Neural Audio: Finding your feet with AI and Audio
- Oberon Day-West
- Mar 5, 2024
- 6 min read
Welcome to our primer series on the dynamic intersect of Artificial Intelligence (AI) and audio technology, a realm many now aptly term "Neural Audio." This field, once predominantly a topic of academic research, continues to be integrated into our everyday personal and professional lives, changing how we engage with and process sound.
This series will take a high-level look through some underlying technology that powers applications that can classify audio and music, handle speech input and classification, and show how this might eventually be utilised in game audio applications and pipelines. We will outline the topic of Neural Networks and Deep Learning and how these are at the forefront of innovative audio design and music production methods. Finally, we aim to unravel how AI equips the audio industry with tools and review how these developments might shape future applications, particularly in game audio development.
What is AI, Machine Learning, Deep Learning and Neural Networks?
Artificial Intelligence (AI): AI, short for Artificial Intelligence, is like giving a computer a bit of human-like thinking power. It's a broad term that covers any task done by a machine that would typically need human intelligence. This could be anything from recognising speech to making decisions. The idea of AI might sound buzzwordy right now, but it's already all around us - think of voice assistants on our phones or intelligent recommendations when we use video services or shop online. AI is a fast-growing field, and what it can do is constantly expanding and under development.
Machine Learning (ML): Machine Learning is a specific part of AI. Think of it as teaching computers to learn from experience. This field is divided into three main types:
Supervised Learning: Imagine we have a bunch of music tracks, and we tell a computer which genre each one belongs to. Supervised Learning is when the computer uses these examples to learn how to identify the genre of new tracks it's never heard before. It's like teaching a student with many examples and testing them on further questions. This is the most widely researched and implemented form of Machine Learning.
Unsupervised Learning: This is a bit different. Here, we wait to give the computer any answers in advance. Instead, we let it look at lots of data and find patterns, associations and structures on its own. For instance, if we give it various voice recordings, it might start noticing which ones are similar in clarity or pitch without us telling it what to look for, and "cluster" the data together.
Reinforcement Learning: In this approach, the computer engages in a process of trial and error, much like learning to play a new game. It improves its performance by understanding which actions lead to success and which do not. Imagine a model or an "agent" that receives a reward when correctly identifying a piano chord and possibly a penalty for an incorrect identification. Over time, these rewards and penalties teach the agent to improve its piano chord recognition abilities. This method is more sophisticated than the previous ones and is predominantly found in academic research, with its presence in real-world applications still growing.

An overview diagram of the main areas of Machine Learning, and how they differ with dataset requirements, uses and their respective deployment scope [1, 3].
Deep Learning (DL): a sophisticated subset of Machine Learning that provides intricate methods for how computers interpret and respond to complex data. At its core, Deep Learning involves a series of computational layers. These layers collaboratively analyse and process input data, enabling the model to make intricate decisions. The more layers a model has, the 'deeper' it becomes, allowing it to discern and learn from increasingly complex patterns. This depth is crucial for handling the multifaceted nature of audio data.
In the context of game audio, consider an immersive environment where audio reacts in real-time to player actions and game scenarios. Deep Learning models could be trained to recognise and differentiate various sound patterns, like footsteps on different surfaces or ambient environmental noises. This could be developed into a system for procedurally generating the appropriate sound effects based on the player's actions, such as changing footstep sounds when moving from a grassy field to a gravel path.
Another significant application is in adaptive music composition. Here, Deep Learning models, fed with various musical compositions, could learn the emotional and contextual impacts of different musical elements in a composition. They could then generate or modify the background music in response to a player's in-game activities or emotional cues.
Neural Networks (NNs): Neural Networks are a big part of Deep Learning. These are complex models designed to mimic how human brains work. Within NNs, there are different types, such as:
Convolutional Neural Networks (CNNs): These are great for tasks that involve images or audio. They can pick out patterns like edges in a photo or specific sounds in an audio clip or spectrogram.
Recurrent Neural Networks (RNNs): These are useful for things that have sequences, like sentences in a language or notes in music. They're good at predicting what comes next in a series.
Each area - AI, ML, DL and NNs - has unique tricks and uses and can take several blog series to explain their inner workings and details. That is out of the scope of this introductory series. However, as we dive deeper into Neural Audio in general, we'll explore how these technologies converge and provide some possible applications for interacting with sound, game audio and music.
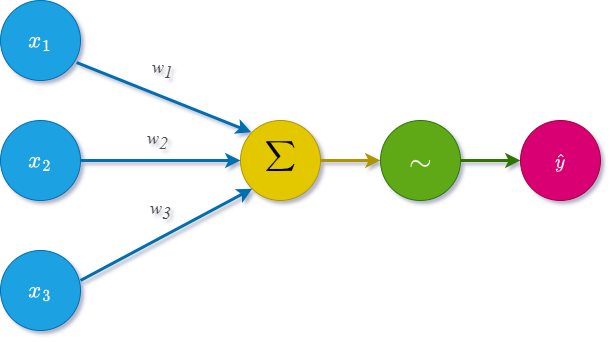
This diagram illustrates a single artificial neuron in a neural network, showing inputs being weighted, summed, passed through an activation function, and then outputted as a single value [1, 3].
The Intersection of AI and Audio
AI's role in audio technology is vast, covering everything from Automatic Speech Recognition (ASR) to Music Generation. This next section will introduce an overview of just a few of these applications.
Music Classification: Consider the popular Shazam app. It captures a snippet of music and compares its waveform against a vast database, seeking a match. This process, known as music classification, is a perfect example of a classification system that is likely continuously being trained on new data to improve its accuracy and dataset.
ASR: Automatic Speech Recognition has become a staple in smartphones and smart speakers. It allows our voices to be transcribed into actions or text, making technology more accessible and responsive. It can also be further developed to assist with accessibility with certain products such as video games or digital audio workstations.
Audio Source Separation: Have you ever wondered how different musical instruments and voices are isolated from a complex audio track? Audio Source Separation is the key. This technology enables the segregation of various elements in a source material, essential for remixing, denoising, audio enhancement or further processing.
Procedural Audio: Some models are also now breaking ground in procedural audio. This involves generating new audio samples based on prompts from the user or system, leading to innovative musical arrangements, patterns and sound effect generation. It's a space where the model can replicate source material and provide additional creative contributions.
Enhancing Game Development with AI Integration
Numerous development teams are actively exploring the integration of machine learning models into the game development process. This integration aims to enhance tooling, systems, conventional AI components, and player interactions. An example is the collaboration between ONNX, a powerful inference engine, and Unreal Engine. The current focus is on developing various plugins that enable the seamless implementation of neural network models, which could offer unique graphic rendering techniques for scenes and various elements within the engine, as depicted below.
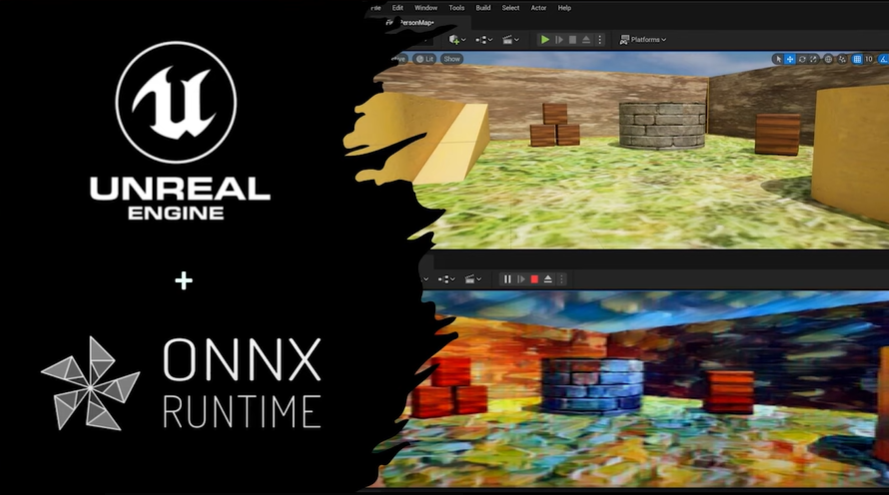
A demo of applying a PyTorch Style Transfer Model that does real time inferencing frame by frame [2].
A note on Ethics in AI
Ethical questions naturally arise as we integrate AI models into our audio design and production pipelines, such as:
Whose data trains these models?
Could they replace human tasks?
What security measures protect intellectual property?
These concerns are hot topics in a collection of focus groups, academic institutions, and policy regulators. Understanding and addressing these issues is crucial for professionals to see AI as a tool that enhances, rather than disrupts, their workflow. As this area is continuously under discussion and review, it is out of the scope of this series to provide clear guidance or opinion on the ethical impact of specific situations.
Up next...
Now that we have laid a universal foundation in some of the terminologies and some possible use cases, we will move on to more Neural Audio-focused topics. Next in this series, we aim to explore Audio Transformers and their multifaceted application in many areas of audio research, development and tooling - stay tuned!
References
Comments